AIOT – Artificial Intelligence Of Things
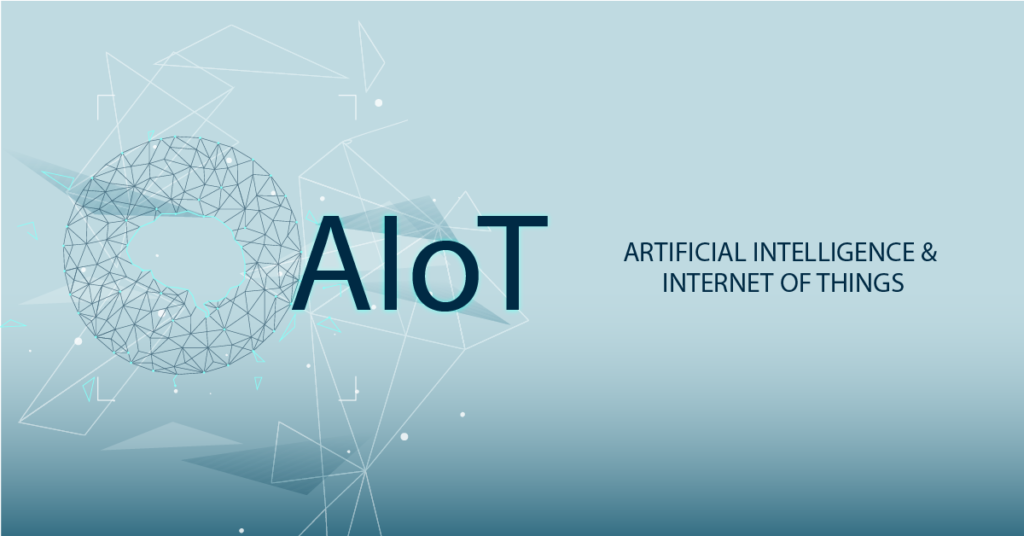
What is AIoT?
Artificial Intelligence of Things or AIoT is the unique combination of two disruptive technologies: artificial intelligence and the internet of things. As the smart internet of things is becoming common in everyday appliances that require intelligence, intuitiveness, and data connections to perform more complex tasks boosted the transformation of electronic devices into intelligence machines for everyday life.
Here is the AIoT overview and general knowledge of AI and IoT, two embracing technology and transformation, three probing technologies with scenario analysis, four connected Hove cloud AIoT solutions.
As IoT and AI technologies become mature, more and more enterprises regard AIoT as their main development direction. Since 2017, the word AIoT has become a buzz in the IoT industry. AIoT is the implementation and integration of AI and IoT in real-life applications. It is not a new technology but a new application form of IoT. If IoT connects all common things that function independently through networks, then AIoT is to provide more intelligent features to connect all things.
In a real sense, AIoT is not simply combining AI and IoT. Ai and IoT technologies are used to integrate data knowledge and intelligence through five G networks based on big data and cloud computing with semiconductors as algorithm carriers and cybersecurity. As implementation assurance, more application scenarios can be implemented and more possibilities can be realized when AI is applied at the application layer. Ai can maximize the value of IoT and IoT can provide data flows required by AI. Only by combining them can the advantages of IoT and AI be maximized.
Ai is a science and technology-based on computer science, neuroscience, mathematics, philosophy, sociology, biology, and psychology. The core innovation force of artificial intelligence is to develop functions inspired by human intelligence similar to interference, learning, and problem-solving. AI is an advanced science-based on studies and theory development, methods, and systems application for simulation and extension of human intelligence. The basic conditions for achieving AI include data algorithms and computing power. Since 2012, the data volume surges, deep learning emerges, and the computing power is improved.
Ai starts to burst explosive data volume growth provides sufficient nutrients for AI. Dc International Data Corporation predicts that the global data volume will reach 40 ZB by 2020, and the data volume in China will reach eight six ZB, accounting for about 21% of the global data volume. In terms of algorithms, the emergence of deep learning breaks through the limitations of shallow learning algorithms used in the past and disrupts the algorithm design ideas in basic application fields such as voice recognition, semantic understanding, and computer vision for computing power.
The emergence of GPU, NPU, and FPGA dedicated chips makes the data processing speed no longer a bottleneck of AI development. Common AI technologies include traditional machine learning, deep learning, and cutting-edge reinforcement learning.
Machine learning is the core of AI. Machine learning is mainly to design and analyze some algorithms that allow computers to automatically learn. These algorithms include decision tree algorithm, particle swarm optimization, PSO algorithm, random forest algorithm logistic regression algorithm support, vector machine, SVM algorithm, genetic algorithm naive Bayes algorithm, K nearest neighbor method greedy algorithm, Kmeans algorithm, AdaBoost algorithm, Ant colony optimization, ACO algorithm neural network algorithm and Markov algorithm. Deep learning is derived from the research search of artificial neural networks and multilayers. Perceptron is a type of deep learning structure.
Deep learning is a new field in machine learning research. Its motivation. This is to establish neural networks that simulate the human brain for analysis and learning, including generative, Adversarial networks, Gan, Convolutional neural networks, CNN, and Recurrent neural networks on reinforcement. Learning is one of the paradigms and methodologies of machine learning. It describes and solves the problems that an intelligent entity maximizes the return or achieves a specific objective by learning policies during interaction with the environment. The main algorithms include QLearning and Monte Carlo algorithms. There are many technical directions of AI, such as statistical learning, data mining, pattern recognition, machine vision, voice recognition, natural language processing, human-machine interaction, and expert systems.
Ai is widely used in our life. For example, voice translation through natural language processing, including feature extraction, pattern matching criteria, and model training. Machines can convert voice signals into texts or commands. Understanding human languages by sentences and their meanings. Machines can now deal with different accents, slang, background noises, and different person stones.
Voice translation is commonly used in the voice input system, voice control system, and Intelligent Query system. Identity recognition can systematically understand and interpret visual input on a computer and accurately identify abnormal people through learning. It can also be used in the clinical expert system to diagnose patients, diseases, and for vehicle license plate recognition. Intelligent robots can perform tasks given by human beings. They have sensors that can detect data from the real world, such as light, heat, temperature, motion, sound collision, and pressure efficient processors inside them.
Working together with multiple sensors and huge memory, demonstrate their intelligence and allow them to learn from mistakes to adapt to the new environment for expert systems, some applications have been integrated with machines, software, and special information. They provide users with explanations and suggestions. For example, they can analyze the stock market and conduct quantitative transactions. In addition, there are machine vision, fingerprint recognition, iris recognition, palm print recognition, handwriting recognition, automatic planning, intelligent search, theorem proving, gaming, game design, automatic program design, intelligent control, language, and image understanding, and genetic programming.
Ai has undoubtedly been applied in many areas. Iot is an extension and expansion of the Internet. It connects sensors with the Internet to form a giant network implementing interconnection of people, machines, and things anytime, anywhere. The IoT architecture is complex. The IoT construction consists of four parts. The first part is necessary device Hardware The things in the IoT devices serve as interfaces between the real world and the digital world with different sizes, shapes, and technical complexity.